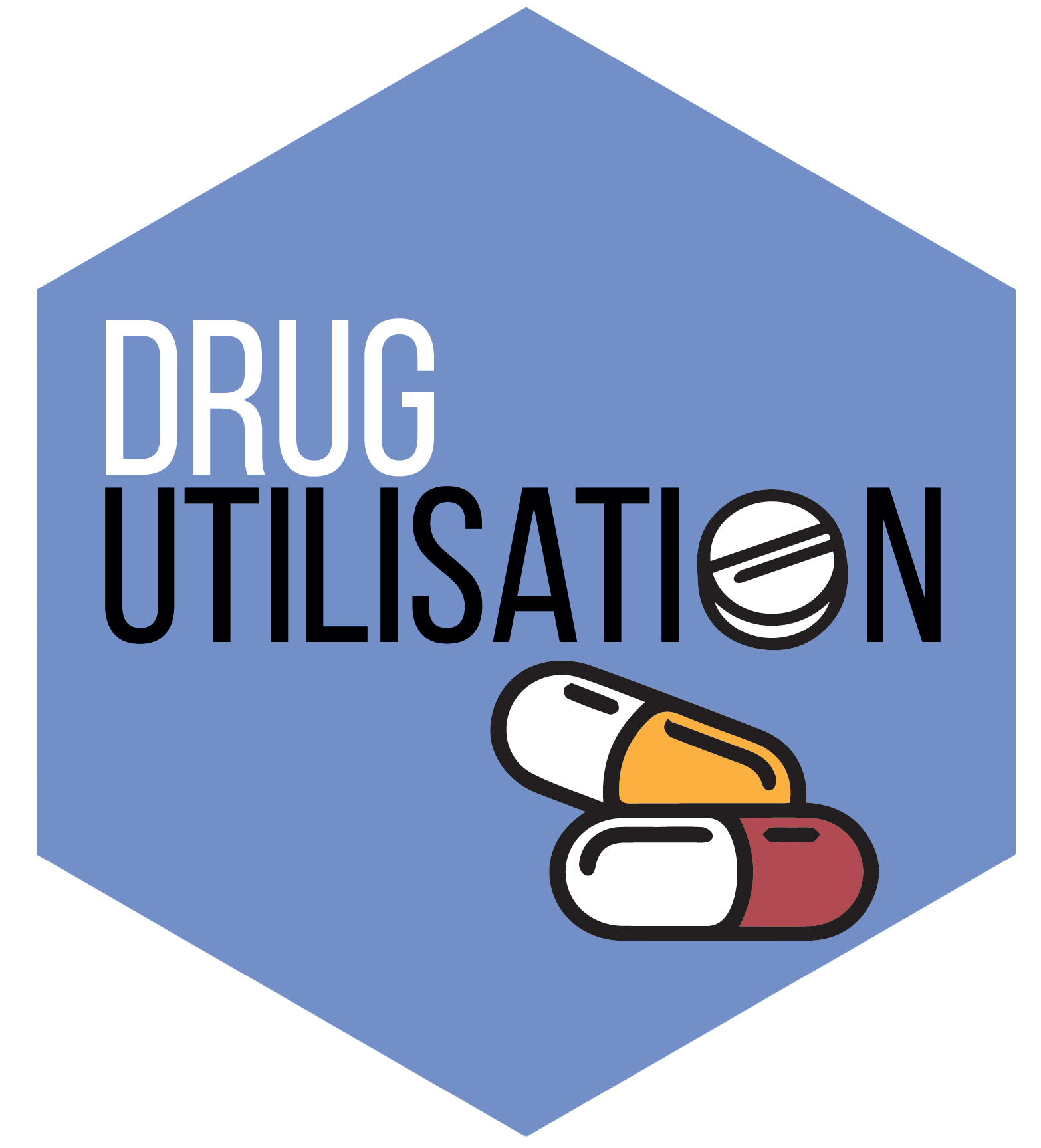
Summarise the indications of individuals in a drug cohort
Source:R/summariseIntersect.R
summariseIndication.Rd
Summarise the observed indications of patients in a drug cohort based on their presence in an indication cohort in a specified time window. If an individual is not in one of the indication cohorts, they will be considered to have an unknown indication if they are present in one of the specified OMOP CDM clinical tables. Otherwise, if they are neither in an indication cohort or a clinical table they will be considered as having no observed indication.
Arguments
- cohort
A cohort_table object.
- strata
A list of variables to stratify results. These variables must have been added as additional columns in the cohort table.
- indicationCohortName
Name of the cohort table with potential indications.
- cohortId
A cohort definition id to restrict by. If NULL, all cohorts will be included.
- indicationCohortId
The target cohort ID to add indication. If NULL all cohorts will be considered.
- indicationWindow
The time window over which to identify indications.
- unknownIndicationTable
Tables in the OMOP CDM to search for unknown indications.
- indexDate
Name of a column that indicates the date to start the analysis.
- mutuallyExclusive
Whether to report indications as mutually exclusive or report them as independent results.
- censorDate
Name of a column that indicates the date to stop the analysis, if NULL end of individuals observation is used.
Examples
# \donttest{
cdm <- mockDrugUtilisation()
indications <- list("headache" = 378253, "asthma" = 317009)
cdm <- CDMConnector::generateConceptCohortSet(cdm, indications, "indication_cohorts")
cdm <- generateIngredientCohortSet(
cdm = cdm, name = "drug_cohort",
ingredient = "acetaminophen"
)
#> ℹ Subsetting drug_exposure table
#> ℹ Checking whether any record needs to be dropped.
#> ℹ Collapsing overlaping records.
#> ℹ Collapsing records with gapEra = 1 days.
cdm$drug_cohort |>
summariseIndication(
indicationCohortName = "indication_cohorts",
unknownIndicationTable = "condition_occurrence",
indicationWindow = list(c(-Inf, 0))
) |>
dplyr::glimpse()
#> ℹ Intersect with indications table (indication_cohorts)
#> ℹ Summarising indications.
#> Rows: 12
#> Columns: 13
#> $ result_id <int> 1, 1, 1, 1, 1, 1, 1, 1, 1, 1, 1, 1
#> $ cdm_name <chr> "DUS MOCK", "DUS MOCK", "DUS MOCK", "DUS MOCK", "DUS …
#> $ group_name <chr> "cohort_name", "cohort_name", "cohort_name", "cohort_…
#> $ group_level <chr> "acetaminophen", "acetaminophen", "acetaminophen", "a…
#> $ strata_name <chr> "overall", "overall", "overall", "overall", "overall"…
#> $ strata_level <chr> "overall", "overall", "overall", "overall", "overall"…
#> $ variable_name <chr> "Indication any time before or on index date", "Indic…
#> $ variable_level <chr> "asthma", "asthma", "headache", "headache", "asthma a…
#> $ estimate_name <chr> "count", "percentage", "count", "percentage", "count"…
#> $ estimate_type <chr> "integer", "percentage", "integer", "percentage", "in…
#> $ estimate_value <chr> "0", "0", "1", "12.5", "1", "12.5", "0", "0", "6", "7…
#> $ additional_name <chr> "window_name", "window_name", "window_name", "window_…
#> $ additional_level <chr> "-inf to 0", "-inf to 0", "-inf to 0", "-inf to 0", "…
# }